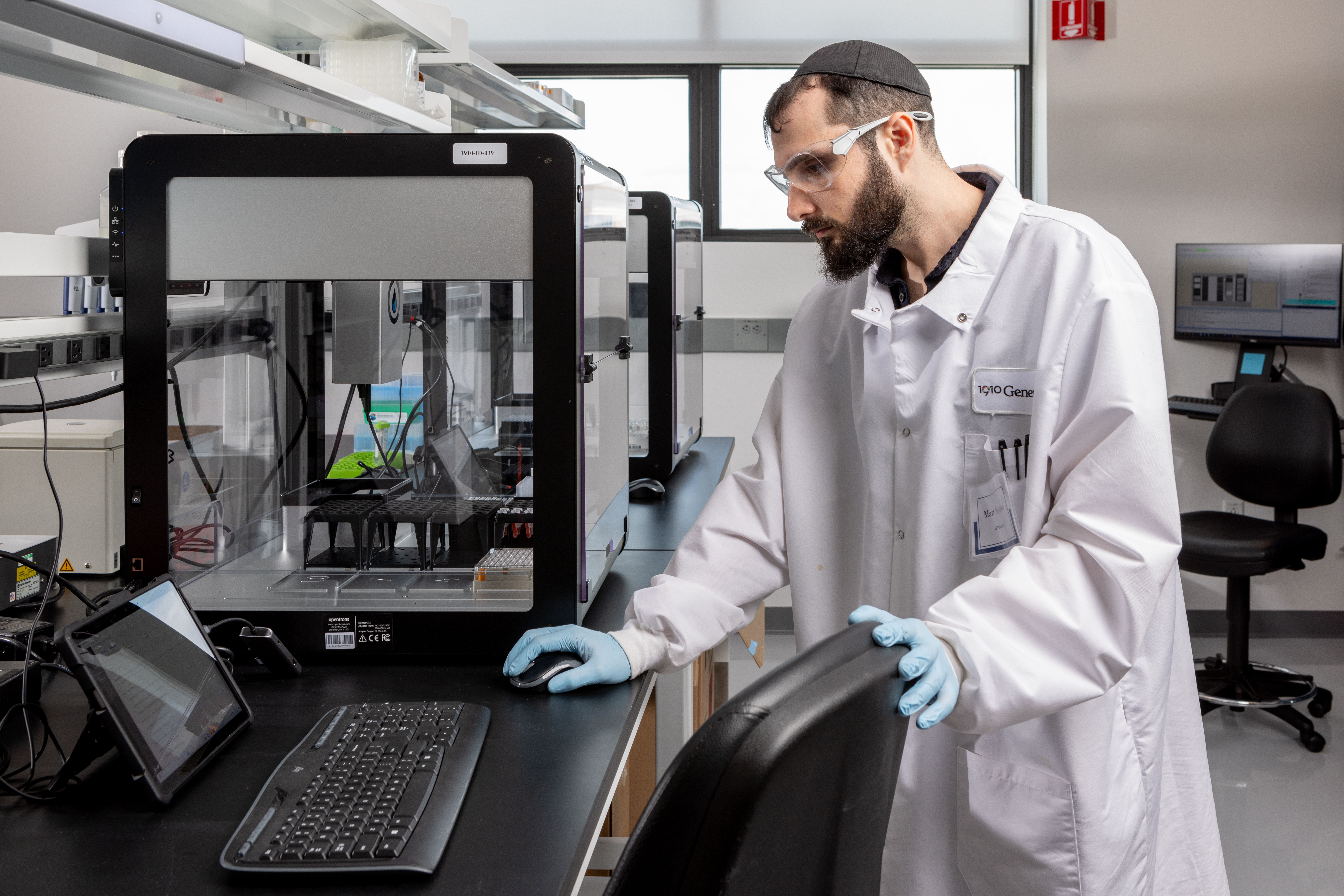
Our Input-Transform-Output (ITO™) platform addresses key challenges of small & large molecule drug discovery
input
transform
output
Modality Agnostic
ITO™
Platform
We address key challenges of small & large molecule drug discovery with our Input-Transform-Output (ITO™) platform.
Large Molecules
- Peptides
- Monoclonal Antibodies
- VHHs
- ADCs
- Fabs
- ...and more
Small Molecules
- Traditional Small Molecules
- Covalent Inhibitors
- Protein Degraders
- Protein Stabilizers
- ...and more
- Precision Neuroscience
- Autoimmune & Immunology
- Precision Oncology
- Rare & Genetic Diseases
- Infectious Diseases
We offer a variety of engagement models.
- Co-Discovery Partnerships
- Co-Engineering Partnerships
- Platform-as-a-Service
- Asset Licensing
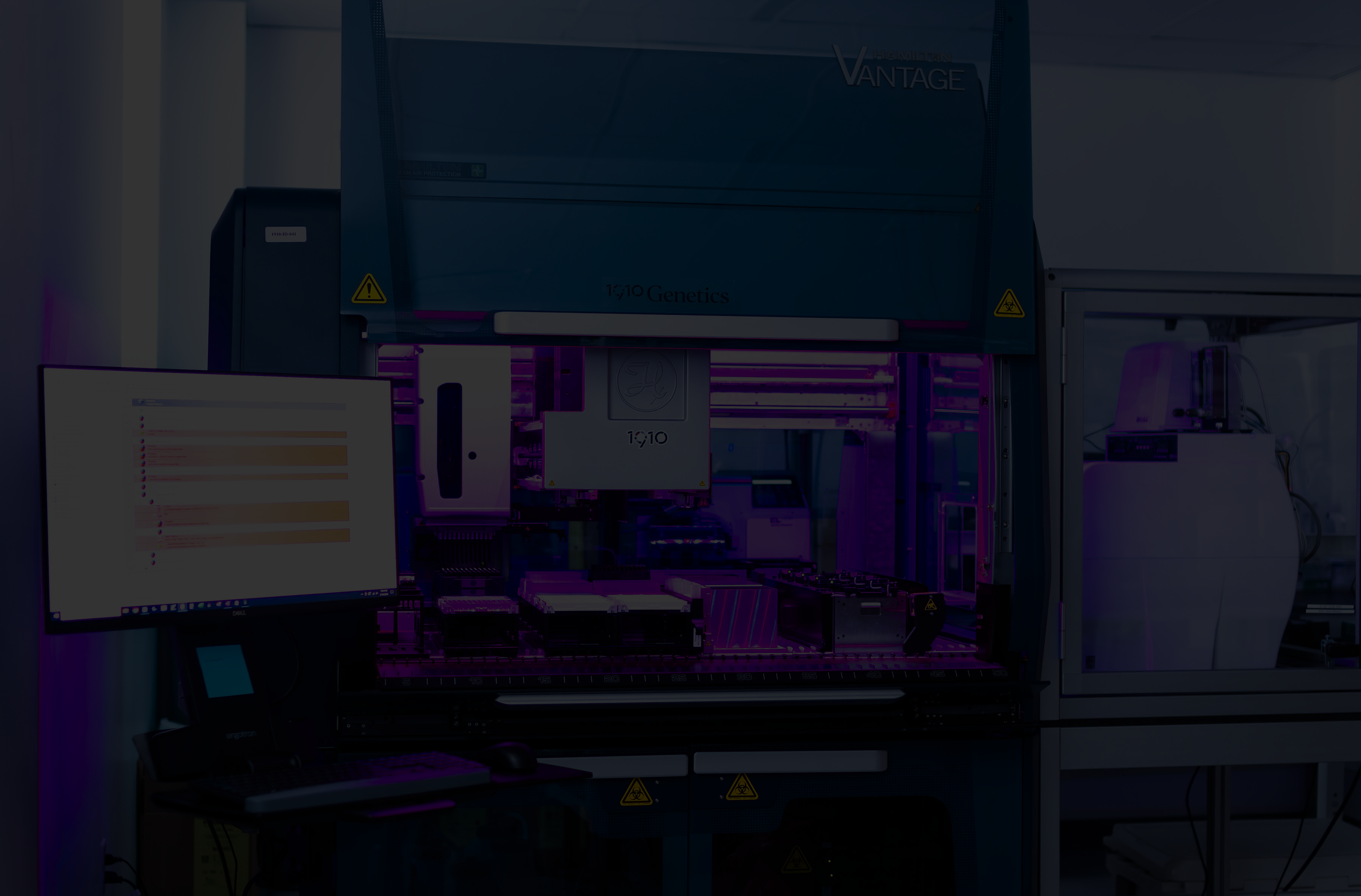
Partnering
Our unprecedented collaboration with Microsoft will build the most powerful, fully-integrated, AI-driven drug discovery and development platform to dramatically improve pharmaceutical R&D productivity, and bring novel therapeutics to patients faster and more cost-effectively than traditional approaches.
learn more